SparseBTF: Sparse Representation Learning for Bidirectional Texture Functions
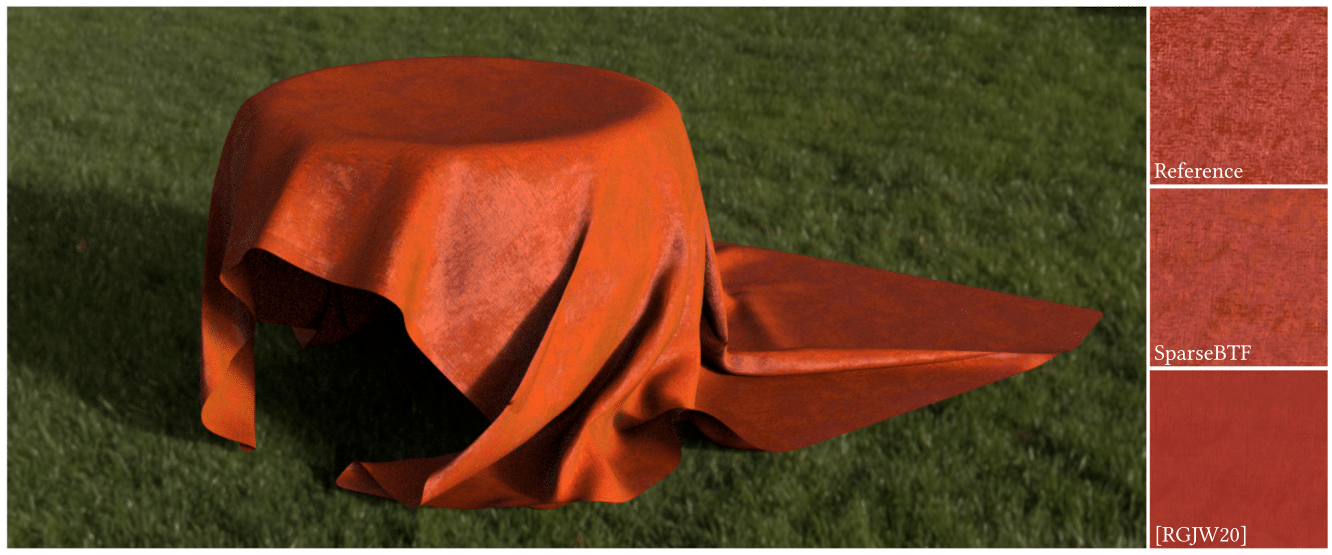
Conference or journal: Eurographics Symposium on Rendering
Date : 2023.06
Kavoosighafi, Behnaz and Frisvad, Jeppe Revall and Hajisharif, Saghi and Unger, Jonas and Miandji, Ehsan
We propose a novel dictionary-based representation learning model for Bidirectional Texture Functions (BTFs) aiming at compact storage, real-time rendering performance, and high image quality. Our model is trained once, using a small training set, and then used to obtain a sparse tensor containing the model parameters. Our technique exploits redundancies in the data across all dimensions simultaneously, as opposed to existing methods that use only angular information and ignore correlations in the spatial domain. We show that our model admits efficient angular interpolation directly in the model space, rather than the BTF space, leading to a notably higher rendering speed than in previous work. Additionally, the high quality-storage cost tradeoff enabled by our method facilitates controlling the image quality, storage cost, and rendering speed using a single parameter, the number of coefficients. Previous methods rely on a fixed number of latent variables for training and testing, hence limiting the potential for achieving a favorable quality-storage cost tradeoff and scalability. Our experimental results demonstrate that our method outperforms existing methods both quantitatively and qualitatively, as well as achieving a higher compression ratio and rendering speed.
Full article link here.